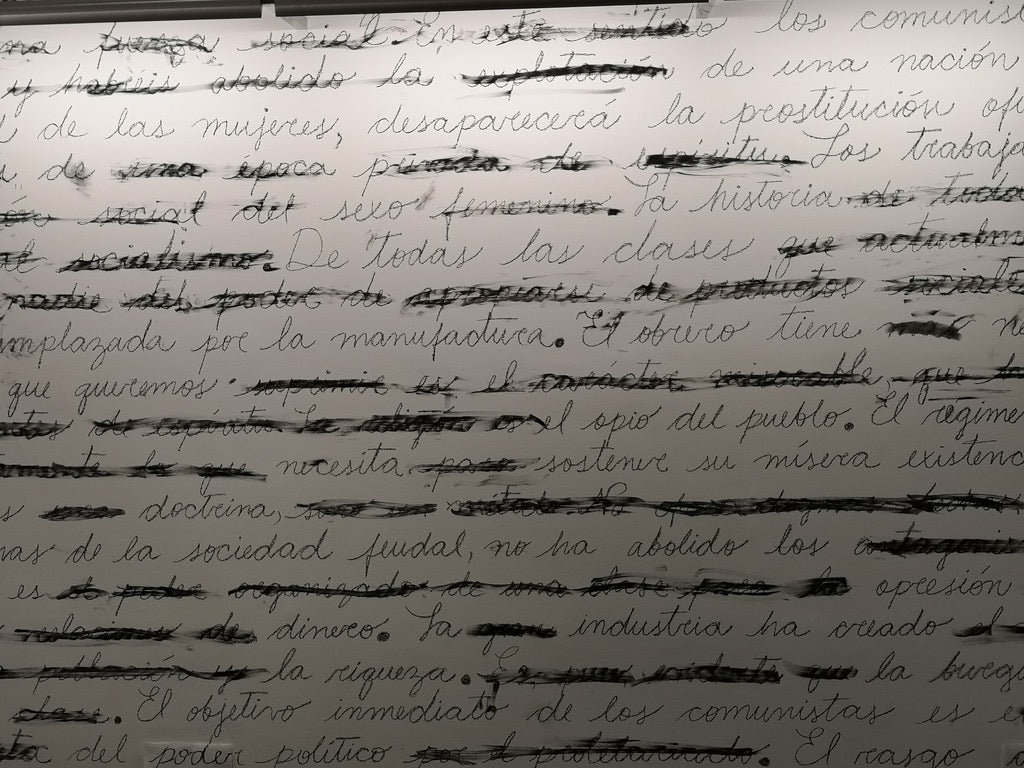
Critique in the Arts: Point versus System
Share
Today, we discuss the potential for AI to either consolidate power or to decentralize control, thereby enabling individual decision-making. This pivot hinges on AI's ability to separate prediction from judgment. Lower costs of decisions can diminish the need for rigid institutional structures and promote a more organic decision-making process. We also look at how institutions use objectification mechanisms to handle complexity, a process that can stifle adaptability and access to information. Furthermore, we borrow the distinction between 'point solutions' and 'systemic solutions' in the context of AI applications in the arts. While point solutions focus on addressing specific tasks, systemic solutions aim at widespread transformation. Often these two types of solutions are bundled together in AI critique and we are curious if class interests play a role in why. We need to unpack the ethical implications of these point solutions but should be cautious about disregarding system-level solutions as a result. Those driving critique often have a vested interest in maintaining institutional systems as they are.
AI and Prediction
We are very sympathetic to anarchist thought. Our approach to AI is shaped by this sympathy, in particular the belief that modern Western institutions are, after Ivan Illich, a ‘corruption’ of our ability to turn toward each other. Our excitement around AI extends from a belief that AI has the potential to de-centre institutional thinking and action. Of course, it also has the potential to centralize control in service to ambitions of efficient coordination, but which direction things will go feels like an open question for the moment, at least.
Institutions have grown large and rigid in part due to the challenges of dealing with complexity. Rules and repeatable patterns of behaviour allow us to move forward despite our ignorance. We believe that the decoupling of prediction and judgment by AI in decision making will reduce the need for ossified structures. This decoupling also has the potential to move decisions back to individuals at points of contact with the world around them.
Making a decision, whether about business strategy or dinner plans, involves both prediction and judgment. We consider the options and make our best guesses about which will lead to an optimal outcome. Our values and the values of the culture around us inform the size and shape of what an ‘optimal’ outcome looks like.
Artificial intelligence is principally about prediction – the next word in a sentence, precipitation levels on farmland impacted by climate change, a movie I might want to watch. In Power and Prediction, Ajay Agrawal, Joshua Gans, and Avi Goldfarb argue that AI’s primary impact will be on decoupling prediction from judgment. The implications of this are significant.
Traditionally, prediction and judgment were closely intertwined. Humans would gather information, analyze it, and make decisions based on their judgment and experience. However, with the rise of AI and machine learning algorithms, the ability to make accurate predictions has become increasingly automated.
AI systems excel at processing vast amounts of data, identifying patterns, and making predictions based on those patterns. They can uncover insights and trends that humans might miss, enabling groups of people to make data-driven decisions with greater precision and efficiency. This decoupling allows for predictions to be made without relying solely on human judgment.
The Growth and Ossification of Institutions
In a world where the cost of prediction is high, we cope by developing repeatable patterns of action, behaviour, and thought. We reduce the complexity of incidents to a tolerable, manageable level. These patterns take the form of habits, rituals, modelled behaviours, expectations, prejudices, meaning constructs, and worldviews. The process of reduction makes events seem more predictable.
The inner life of institutions is controlled by the reduction of complexity and the institution maintains this control through shared symbols, hierarchies of value and vision, customs, rituals, role assignments, structural hierarchies, and above all through the objectification of agreements.
Objectification of consensus and difference takes place both through the construction of artificial structures (infrastructure, legal codes, walls, etc.) as well as through internalization (habits, perception and expectation patterns, behaviour, prejudices, etc.). Objectification allows for permanent distinctions to be made; what is important or unimportant, useful or useless, permissible or prohibited, desirable or undesirable, true or false and so on.
Making such distinctions means making selections and, from a system-theoretical point of view, can then be understood as information. Put another way, as the system selects from all the noise an element and assigns it a specific meaning, the specific element becomes defined and formed – it has importance for that system and thereby becomes information for ongoing self-referential operations. As Gregory Bateson offered, “information is a difference that makes a difference”.
There is a constant tension between the ongoing development of an institution and its desire to harden its internal (reified and internalized) structures. As institutions interact with other systems in a dynamic environment, reductions of complexity can hinder or prevent the survival, adaptability and learning of the system. Objectification not only limits the available behaviours that an organization can deploy to adapt to its environment, but also the information that it can make use of in decision making and other organizational activities.
Implications for the Arts
The current debate about AI and the arts tends to focus on point solutions rather than system-level transformations.
Point solutions refer to narrow applications of AI that address specific problems or tasks. These solutions are often designed to automate or optimize particular processes within a given context. Point solutions in the arts might include replacing human artists with AI-generated images or using AI algorithms to automate routine customer service inquiries.
On the other hand, systemic solutions encompass broader, more comprehensive approaches that aim to address larger-scale challenges or transform entire systems. Systemic solutions involve integrating AI and ML into the core operations and decision-making processes of organizations or industries. Rather than focusing on isolated tasks, systemic solutions seek to leverage AI across multiple functions, departments, or sectors to drive fundamental changes.
The challenge of systemic solutions is that they impact all of the “habits, rituals, modelled behaviours, expectations, prejudices, meaning constructs, and worldviews” accumulated over time. They often involve rethinking business models, redesigning processes, and fostering cultures of innovation and continuous learning.
We should be critiquing point solutions of AI. However, conflating point solutions with potential system-level applications can serve to obscure existing patterns of power and self-interest. Both large arts institutions and universities are hotbeds of critique of AI’s application in the arts. The critique, overwhelmingly, is either existential or focused on misaligned point solutions.
To reiterate, this critique is necessary and important.
However, we might also want to map out the overlap between one’s individual economic, social, and class interests and our desire to preserve the institutional systems on which these interests rely.
AI holds the potential to deliver art in ways previously unimaginable and to provide education that is affordable, learner-driven, and local. Those benefiting from large, centralized arts institutions or massive, capital-intensive universities certainly have legitimate critiques of how AI is being applied. However, we should disentangle concerns about local applications of AI from class interests in the maintenance of existing systems and institutional structures.
We support workers that seek to prevent the automation of their work. We prefer that we are honest about our intentions, though. A professor for an MFA program may very well be concerned about the replacement of artists with machines, but if by leveraging their moral and academic expertise, they also happen to resist the restructuring of their place of employment, then we should be open about the entangled nature of the various interests at play.
The arts has a long history of resisting fundamental changes, because those tasked and funded to produce art have a vested interest in how art is made and delivered in any given period. By presenting critique as a pure application of expertise, we lose touch with how existing institutional structures shape support and resistance to system-level transformations.
Let me know in the comments how this feels. What would AI-driven system-level change in the arts look like? How might we productively critique AI while still pushing for a fundamental reorganizing of society?